Eric Siegel is a consultant, speaker, and former Columbia University professor. He is the founder of the long-running Machine Learning Week conference series and executive editor of The Machine Learning Times. At Columbia, he won the Distinguished Faculty award when teaching graduate computer science courses in machine learning and artificial intelligence.
Below, Eric shares five key insights from his new book, The AI Playbook: Mastering the Rare Art of Machine Learning Deployment. Listen to the audio version—read by Eric himself—in the Next Big Idea App.
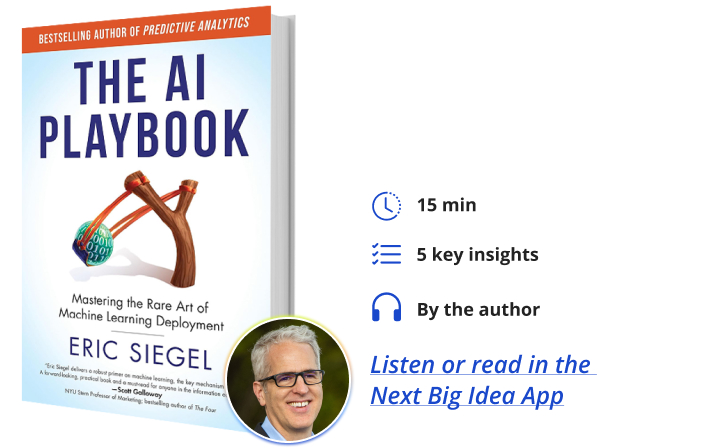
1. The world loves machine learning too much—which actually impedes its deployment.
We fetishize the core technology—the awesome capability to learn from data—which distracts from the rigorous, practical planning needed to get it launched. We’re enamored with how it works, the very idea of it, rather than what it will do for you, the value.
Yes, machine learning is the coolest. It’s the world’s most important general-purpose technology. It can improve almost any large-scale process. But in a way, it’s become too hot for its own good. For all the hoopla about it—often branding it as “AI”—the gritty details of how its deployment improves business operations are often glossed over.
The hype misleads people by feeding a common misconception called The Machine Learning Fallacy. Here’s what people mistakenly believe: since machine learning algorithms work—they succeed in generating predictive models that hold up for new, unseen situations (which is amazing and true)—the models they generate are intrinsically valuable (not true).
The value of machine learning comes only by launching it to enact organizational change. After generating a model with machine learning, you capture its potential value only when you deploy it so that it actively improves operations. Until a model is used to reshape how your organization works, it is literally useless. A model doesn’t solve any business problems on its own, and it isn’t going to deploy itself. Machine learning can be the disruptive technology it’s cracked up to be, but only if you disrupt with it.
Unfortunately, businesses often fail to bridge the biz/tech “culture gap,” a disconnect between data scientists and business stakeholders. Many business professionals are inclined to forgo the particulars as “too technical”—partly because they’ve been seduced into seeing this stunning technology as a panacea that solves problems on its own. They defer to data scientists for any project specifics. But when they’re ultimately faced with the operational change that a deployed model would incur, they’re taken off-guard and hesitate to alter operations that are key to the company’s profitability.
As a result, only 22 percent of data scientists say that their models developed to enable a new process or capability usually deploy. It’s so ironic: People tend to focus more on the technology than how it should deploy, which is like being more excited about the development of a rocket than its launch!
2. Backward planning is key to a successful machine learning launch.
The first step of the bizML practice is to plan precisely how machine learning will be deployed to improve operations. This is a simple and yet surprisingly underutilized trick of the trade.
A glowing example comes from UPS. The company used machine learning to streamline its delivery of 16 million packages a day. This use of machine learning is a key component of an optimization system—that also prescribed driving routes—which annually saves the company 185 million miles of driving, 8 million gallons of fuel, $350 million, and 185,000 metric tons of emissions!
“The company used machine learning to streamline its delivery of 16 million packages a day.”
This tremendous win came not just from advanced number crunching but only when this century-old company was able to change its established ways. That’s machine learning deployment, the culminating step of a machine learning project where predictions take action and make a measurable difference in everyday operations.
The leader of this initiative, a man by the name of Jack Levis, not only led the idea formation and number-crunching, but he also tackled a much greater challenge: implementing big operational change. He had to convince executives and, by way of a change management team that grew to 700 people, he also had to train great numbers of personnel working on the loading docks and driving the delivery trucks to follow new procedures that were built on the predictions generated with machine learning.
What exactly does UPS predict with machine learning and why?
3. Business professionals must upskill on a semi-technical understanding.
To deeply collaborate on and make critical contributions to machine learning projects, all stakeholders need to ramp up on some surprisingly accessible and totally fascinating know-how. This means getting your hands a bit dirty. You can’t just say, “Let’s throw awesome technology at this problem.” You need to get concrete about how the predictions that machine learning delivers will actively improve operations.
In a nutshell, here’s what you need to know about each project: What’s predicted, how well, and what’s done about it. For example, UPS improved its system that assigns packages to delivery trucks by predicting which destination addresses will receive a package tomorrow. These predictions augment the list of known deliveries so that a more optimal overall plan across trucks and packages can be carried out, loading the trucks overnight for their departure in the morning.
“This kind of data literacy is for everyone—it’s like driver’s education, not auto-mechanic school.”
The thing is, stakeholders have to concern themselves with the gory details in order to help guide the project to a successful launch. This includes the full specifics about exactly what the project should predict. For UPS, this came down to, for each destination, how many package deliveries across how many truck stops will be required. For example, “The group of three office buildings with 24 business suites at 123 Main St. will require two stops with three packages each by 8:30 a.m.”
This kind of data literacy is for everyone—it’s like driver’s education, not auto-mechanic school. Once you’ve ramped up, more than anything, non-data scientists like you are exactly what machine learning projects need.
Another example covered in the book is FICO, which has developed a predictive model used to screen all transactions for 2.6 billion payment cards worldwide—that’s two-thirds of the world’s cards, including about 90 percent of those in the US and the UK. For each card transaction, it predicts whether it’s fraudulent. What’s done about each prediction? Your bank’s system automatically decides, instantaneously, whether to allow or hold your payment.
4. If you aren’t measuring value, you’re not pursuing value.
Once you’ve established what machine learning will predict, the next question is how well it predicts. Fortunately, evaluating its performance doesn’t require becoming a technical expert since you can benchmark a machine learning model without regard to its inner workings. We can judge how well it predicts without necessarily judging how it predicts.
You will often hear of accuracy, a simple tally of how often a model predicts correctly. But accuracy is not only the wrong measure for most machine learning projects; it also feeds a common fallacy that tremendously mismanages expectations. Models don’t generally predict anywhere near as well as a perfect magic crystal ball—so what kind of meaningful, useful number can you put on how well models predict? There are two ways.
The first, which is important to data scientists, is with technical metrics such as accuracy (often misleading), and a more useful one called lift, which is a kind of “predictive multiplier” (how many times better than guessing does it predict)? There are many such metrics, and they’re helpful to data scientists for quantifying a model’s relative predictive performance, but they’re insufficient because they provide no direct reading on the absolute business value a model could deliver.
“Technical metrics dominate the machine learning practice.”
The other—which is important to business-side stakeholders—is with business metrics, aka key performance indicators, such as profit, savings, ROI, and number of customers saved. These are straightforward for any stakeholder and relate directly to business objectives. They tell you the true value of the imperfect predictions machine learning delivers.
However, it turns out that technical metrics dominate the machine learning practice. They’re pretty much the only kind of metric that most data scientists are trained to work with. This causes a deadly disconnect that leaves stakeholders lacking visibility into the value. They have no meaningful read on how good a model is! Since they can’t make an informed decision on authorizing deployment, this often derails the project entirely.
Although bridging this divide is rare, it’s a surmountable challenge—just make sure you get a data scientist committed to doing so. Once these best practices are in place, the stars align for deployment—but in addition to ensuring the project’s business performance is good, make certain that your deployment is also doing good.
5. We must take on responsible machine learning as a form of social activism.
When you use machine learning, you’re not just optimizing models and streamlining business. You’re governing. In effect, models embody and implement policies that control access to opportunities and resources, such as credit, employment, housing—and even freedom, when it comes to arrest-prediction models that inform parole and sentencing. Insurance risk models determine what each policyholder must pay, and targeted marketing determines who gains discounts, exclusive deals, and even the awareness of certain financial products.
When ML acts as the gatekeeper to these opportunities, it can perpetuate or magnify social injustice, adversely affecting underprivileged groups by undeservingly denying access disproportionately.
For starters, I advocate for the following standards, which are necessary but not sufficient: Prohibit discriminatory models—that is, don’t allow models to make or influence decisions based even in part on protected classes like race and ethnicity. Ensure that costly errors—where the system makes a wrong decision that disadvantages an individual—do not take place more for one protected group than another. Also, advocate for a person’s right to explanation for algorithmic decisions, at least in the public sector.
The thing is, companies generally refuse to take a stand on issues like these. They’re mostly frozen by the vacuous cosmetics demanded by corporate public relations. It’s often only to posture when firms call for machine learning deployment to be “fair, unbiased, accountable, and responsible.” These are vague platitudes that don’t alone guide concrete action. Declaring them, corporations perform ethics theater, protecting their public image rather than protecting the public. Rarely will you hear a firm come down explicitly on one side or the other for the aforementioned standards, for example.
This means that the fate of the millions of people affected by machine learning rests in the hands of individuals and proactive leaders to ensure that this technology is deployed responsibly. By establishing ethical standards as a form of social activism, we can take a stand that makes a positive difference rather than only conveying vague platitudes.
To listen to the audio version read by author Eric Siegel, download the Next Big Idea App today:
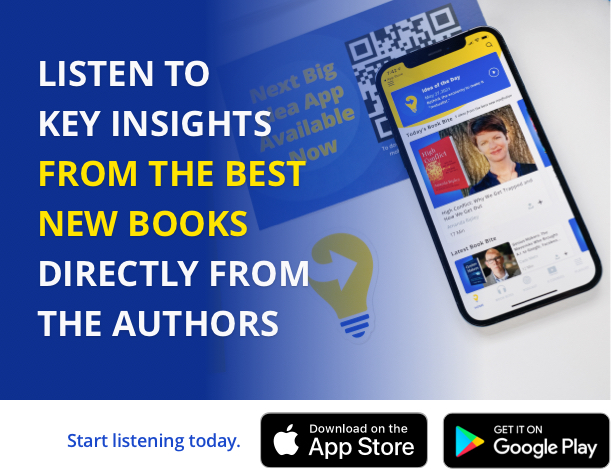