David Auerbach is a writer and software engineer who worked at both Google and Microsoft after graduating from Yale University. He has previously authored a column in Slate and writes on a variety of subjects, including social issues and popular culture, the environment, computer games, philosophy, and literature. His writing has appeared in the Times Literary Supplement, MIT Technology Review, Tablet, The Daily Beast, and Bookforum, among many other publications.
Below, David shares five key insights from his new book, Meganets: How Digital Forces Beyond Our Control Commandeer Our Daily Lives and Inner Realities. Listen to the audio version—read by David himself—in the Next Big Idea App.
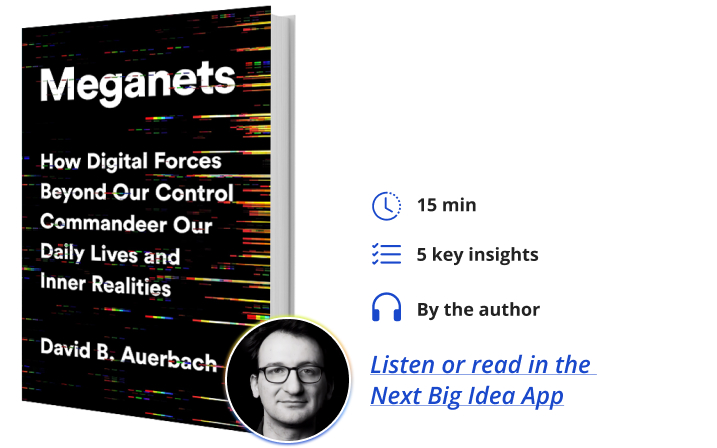
1. We fundamentally misunderstand how online life works, and our control of it.
We are asking the wrong questions about our online lives because we fundamentally misunderstand how computer networks function in today’s world. Software and algorithms have historically been boxed up products made by a technology company. However, for our huge networked systems today, like Facebook, Twitter, Google, Amazon, Bitcoin, Fortnite, and ChatGPT, it’s no longer the case. With these systems, it’s impossible to step into the same data stream twice. Just by participating in these systems, users change the underlying algorithms and data that shape them. The algorithms aren’t the elegant human creations of yesteryear, but elaborate messes of computer-generated spaghetti which cannot be summarized, controlled, or even fully understood.
They are constantly in flux because every one of us exerts a little bit of collective authorship over them. When each one of us has a little bit of power, that results in the creators and operators of these systems having significantly less. Centralized control of these systems is no longer possible. That’s why we feel and see an increasing amount of chaos that no one seems to be able to do anything about. These systems are organic and evolving, not static and precise. They are closer to economies, to the weather, to ecosystems, than they are to classic algorithms and software. We need a new word for these systems; they aren’t only machines or only humans but the combination of the two working together at blinding speed. They can be called “meganets,” persistent, evolving, and opaque data networks that control how we see the world.
2. A meganet consists of both human and machine pieces.
Meganets come about from the combination of two pieces: millions upon millions of users, and a complex algorithm, with AI-driven servers that interact with it. These two components operate with each other in a feedback loop that is too big and fast to contain. There are three defining characteristics of a meganet: volume, velocity, and virality.
“Virality is the feedback effects by which changes lead to more changes.”
Volume refers to the size: the petabytes and exabytes of data that shape these systems. Velocity is the speed at which hundreds of millions of people interact with these systems, instantly updating and transforming the data and algorithms. Virality is the feedback effects by which changes lead to more changes, each change potentially causing a huge ripple effect far beyond its origin. Not all meganet activity goes viral in this way, but with size and speed in play, it’s impossible to predict what will.
3. Meganets underpin a tremendous variety of systems.
Meganets underpin many seemingly different systems; social media, AI, cryptocurrency, online gaming or government identity systems. We may treat these as independent problems, but in fact they are all plagued by the same loss of control. Whether it’s ChatGPT, Facebook or India’s Aadhaar identity service, unwanted behavior arises when the systems become so large and densely networked.
The question is not if but when some sort of cascading failure will arise and with AI, this process is supercharged. The training data sets for any deep learning AIs, whether LLMs (Language Learning Modules) or something else, are so enormous that it is quite literally impossible to validate that everything fed into ChatGPT is “true”—much less guard against the possibility of it producing false “hallucinations” in its responses.
If a meganet can tolerate a decent degree of failure, if showing irrelevant content on a feed or misclassifying someone demographically is tolerable, then for those applications AIs have much to offer. However, if we need human or better levels of performance, we’re faced with an uncomfortable dilemma. We either need more human labor than exists to verify meganet activity, or else be willing to put up with unpredictable behavior from these systems that combine machine efficiency with human unpredictability.
4. We need to see meganets for what they are.
Our failure to understand meganets causes us to look for solutions in the wrong place or worse still, where none exist. We repeatedly ask organizations, whether they’re corporations or government regulators, to take back control of these systems and beat them into the shape we want. Whether we’re begging Facebook to stamp out misinformation or asking Twitter to stamp out abuse or even insisting OpenAI stops ChatGPT from “hallucinating,” we’re making the mistake of thinking that they have control over their systems. This is a misapprehension that many tech companies would prefer us to have, but a false one nonetheless.
“We’re making the mistake of thinking that they have control over their systems.”
In 2020, in response to controversy over a glut of far-right material on Facebook, a Facebook internal executive memo laid out two very important action items: limit the meme that Facebook is slow to spot misuse and can’t control content, and limit the meme that Facebook cannot control its systems or is too slow to spot these different types of abuses.
The reason why they were so concerned with limiting these memes is because they were true. When Facebook banned all political advertising in the run-up to the 2020 election and limited forwarding all links to a maximum of five people at a time, those weren’t policies of a company that exerted micro control over the content in its system. YouTube bans comments entirely on an increasing number of its videos, and that sort of macroscopic move is only necessary because they don’t have fine-grained control.
5. We may not have control, but we can influence and tame them.
While we can’t hope to control meganets in any kind of fine-grained way, there are mitigation tactics for their worst excesses. They’re not cure-alls. We can’t stomp out content we don’t like in an endless game of Whack-a-mole and we can’t anticipate which trends will blow up. However, what we can do is to temper the intense feedback loops that arise with broad, soft, non-targeted ways. Instead of trying to tell “good” from “bad,” which humans can’t do quickly enough and which machines can’t do accurately enough, we can try to soften the feedback-driven excesses.
We can limit the spread of any content that is spreading too quickly, not censoring it but de-ranking it temporarily. We can reduce the volume of the loudest voices and raise it for the quieter voices. We can reduce the strict regime of recommendation algorithms and introduce more randomness and variety in the hopes of breaking up the narrative bunkers that meganets sort people into.
Facebook and TikTok have already tried some of these non-targeted approaches and have met with more success than trying to target specific kinds of content. When it comes to AI, we can exert a lot more care in terms of the selection and filtering of training data. It will take experimentation and coordination to figure out the best way to implement these sorts of mechanisms, but recognizing the limits of our control over meganets is the first step toward actually improving them.
To listen to the audio version read by author David Auerbach, download the Next Big Idea App today:
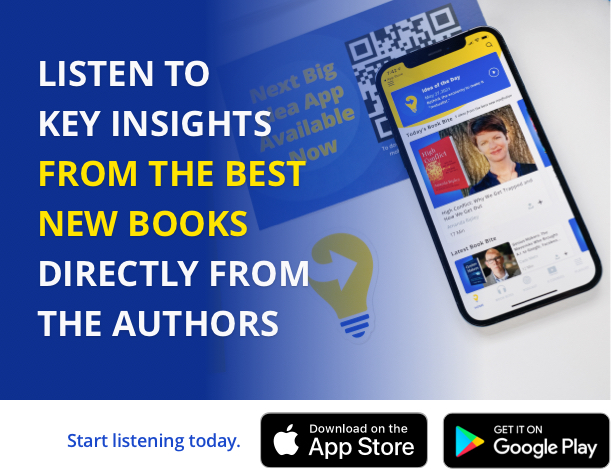